As the potential for the application of Artificial Intelligence grows, there is a lot of hype and excitement that AI can now be a way to more accurately predict risk in large-scale programmes. Is this really true? And how effective could it be?
Programme risk management processes and tools exist to help deliver programmes and projects on time, spec and budget. Finding ways to bring more assurance and rigour to the process and tool is always appealing and it makes good sense to see how AI can take risk management to another level of insight.
Software tools are now emerging that claim to utilise AI to enhance the identification and management of programme risk by collecting lots of data on previous programmes to inform how future programmes might succeed. For example, how long things took, how much they cost, stages where challenges arose etc, which, in theory, all allow AI tools to predict how new programmes will perform.
Indeed, this can be very effective at predicting the future performance of “similar” programmes, for example building a new railway line using conventional methods, by learning from past data on how long things took/cost and what risks emerged and how they were managed, or not. What it cannot do is predict the success of programmes that are dissimilar because it has little or no data or relevant information to learn from. In our example, building a new railway line using robotic equipment that has never been employed before.
Critical areas that bring dissimilarity are innovation and complexity. Introducing innovation into projects brings a level of ‘newness’ that cannot be previously known. For example, using technology that has not been used before. In fact, introducing innovation also leads to complexity in many different forms and complexity also leads to risk. For example, forming large teams across geographies that have not worked together before brings complexity, or operating in a highly political environment where priorities are not clear and may suddenly change etc. For “similar” programmes, AI driven tools would clearly be very effective as there is an abundance of relevant data available. However, complex and/or innovative programmes would clearly be far more difficult to predict and manage using AI as, by definition, relevant data is not readily available.
The use of automation and AI to fuel insights on a far wider and bigger scale is an extremely powerful proposition. The use of AI applied to our SDA methodology can go further than simply capturing data.
A useful article by Gilles Jonk from Management Consultants Kearney recently explained this using a ‘Xerox line’ model, derived from an analogy which considers the photocopier giant’s use of knowledge and information in two stages. That which is below the line is effectively copies or re-used versions of older work. While above the line is where new and truly transformative or original ideas lie. The model is a useful principle to explain how AI in risk management processes might work effectively with the right focus.
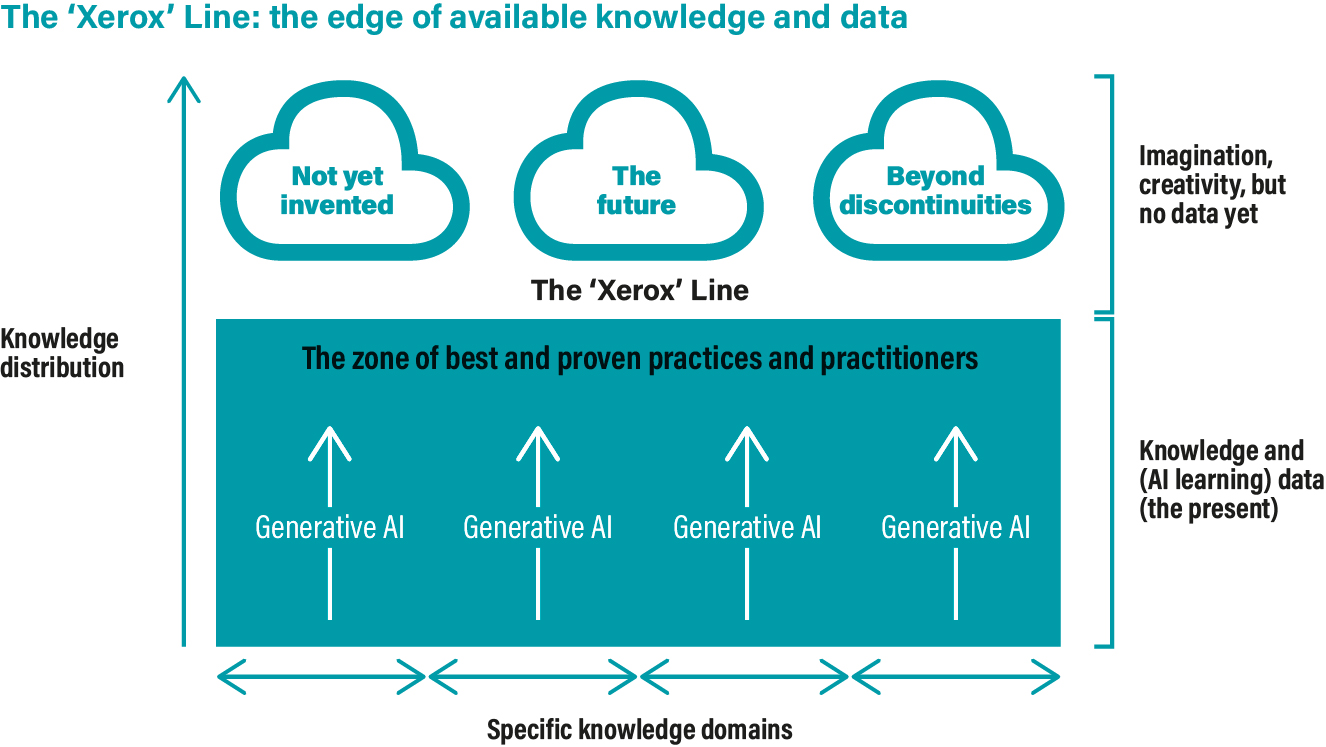
As the Kearney article points out, both domains above and below the line are important to business. From an AI point of view, we can see that information and data points can be gathered from information below the line, meaning that the learning from the past only allows us to “predict” the future in programmes that are similar to previous programmes. So, the more “innovative” the new programme is, AI can get you very close to the Xerox line but not above it.
That’s not to say that AI can’t be incredibly useful in risk management of dissimilar, innovative or complex programmes, but by taking a different focus.
De-RISK is looking at ways that automation AI can further improve our Strategic Delivery Assurance (SDA). Already an extremely effective methodology which brings rigour to large scale, innovative programmes, resulting in more predictability of projects, the use of AI to fuel insights on a far wider and bigger scale is an extremely powerful proposition. The use of automation and AI applied to our SDA methodology can go further than simply capturing data (something other tools are also effectively doing).
SDA uses a rigorous process to capture the collective knowledge in a team looking forward i.e. the estimates they are making regarding the programme, in particular on the more innovative aspects, and then interrogates and cross-references these estimates, rigorously, against the captured assumptions, By using the automation and AI capabilities of the proprietary Assure software used within the SDA process, the AI is able to challenge and capture more insight into the risks of the innovative aspects of the programme and how to manage them.
Automation utilising AI techniques can further facilitate this process by taking the place of trainers to make SDA available to large and multiple organisations by avoiding the need for mass training and roll-out programmes i.e. using the software to enable “training at the point of use”. This combination of automating the SDA process, together with the interrogation and interpretation of the innovative risks, suggesting management strategies, and then rolling the process out across the organisation, gets us firmly above the Xerox line in the most effective way possible.
So, we must be cautious of claims that AI is a panacea for the future of risk management if all we do is crunch masses of historical data of similar programmes. However, if we combine this with the use of AI to help to ‘automate’ the SDA process of identification and management of risk in the innovative and complex aspects of the programmes, then AI can really be the future of risk management.
De-RISK’s SDA methodology is a series of steps to deliver confidence that project timescales and benefits can be met.